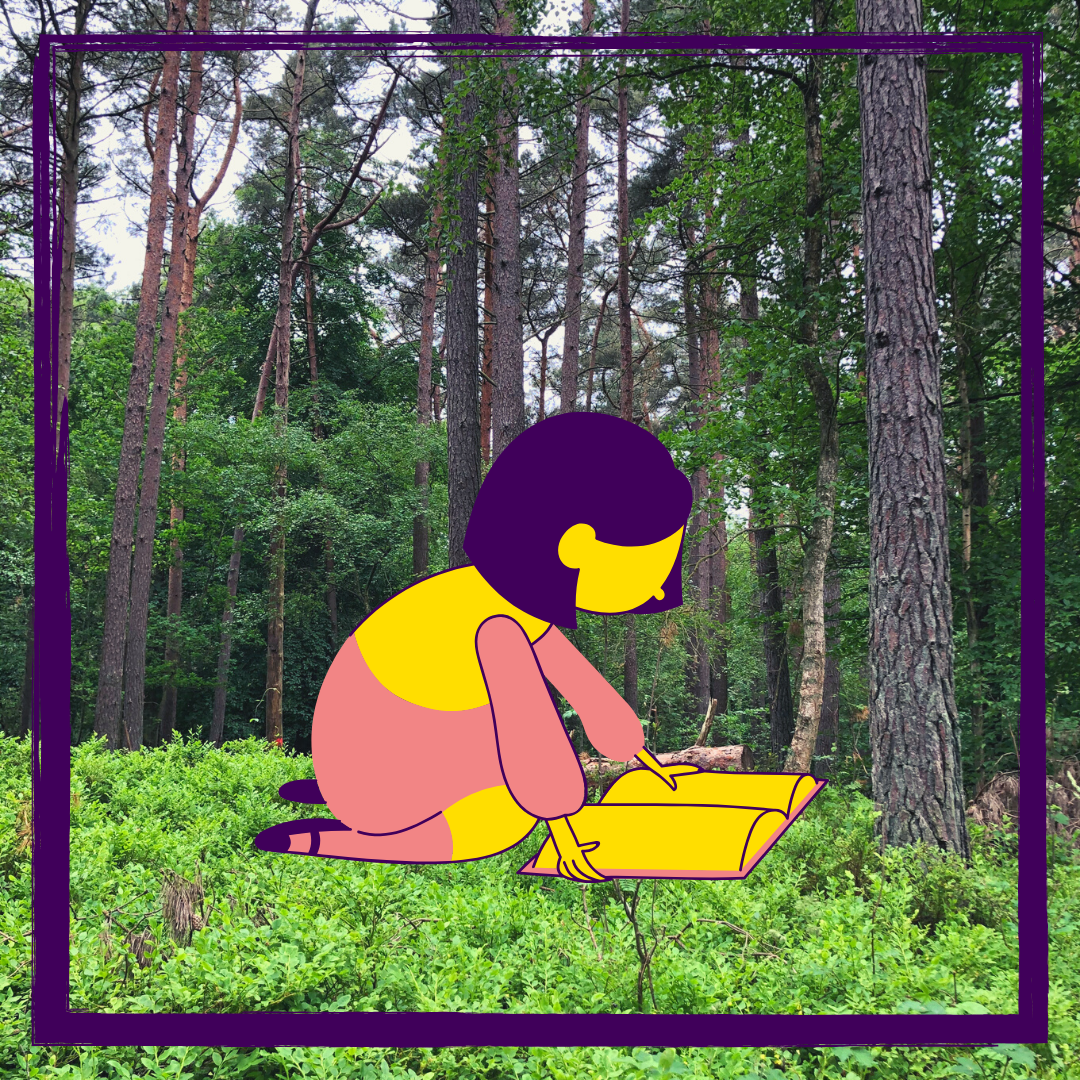
Distant Reading – how much distance can we bear?
Inhalt / Content
In his book “Distant Reading” (Moretti, 2013), which I recently presented here, Franco Moretti deliberately and surely a bit provocatively claims that it would be useless to read more and more. Instead, literary scholars should finally learn the art of not reading. In fact, we now have many digital methods at our disposal with which one can distance oneself from the text, i.e. one does not have to read it oneself, but can use a computer to help. But what inaccuracies must one actually accept if one wants to do Distant Reading? And how can the method be used sensibly? In a self-test, I compared the methods of Close and Distant Reading (here with the help of an “out of the box” named Entity Recognition Tool) with a third variant, casual, not very close reading, let’s call it Quick Reading.
Close Reading
Close Reading is one of the central methods of literary studies. The term refers to the process of reading texts very carefully and interpreting them word for word in terms of deeper semantics. Each word can also be embedded in more than one context of meaning. Very closely related to close reading is the technique of annotation (Jacke, 2018). This is because literary readers often use colour markers to integrate categories of meaning into the text or write annotations in the margin. Close Reading can be carried out both with analogue texts and digitally. Annotation tools such as CATMA (Schumacher, 2019) or WebAnno (Schumacher, 2018) are suitable for digital close reading.
The Close-Reading Self-Test – the framework conditions
For my method comparison, I carefully annotated the first 100 pages of a novel (effectively the first 90 pages, since the text did not begin on page 1) using the CATMA annotation tool. CATMA, by the way, is a web application for literary studies that can be used freely in any browser. Similar to underlining a book with markers, you can use it to create annotation categories and mark text passages with them. However, unlike markers, you can make quantitative queries at the end of the process and visualize the resulting data in graphics. Yes, in CATMA you can even annotate together, as I have already reported here.
The Close-Reading Self Test – the comparison category
In order to have a well comparable category that I can use not only for close reading, but that is also covered by tools for automatic annotation of texts, I decided to annotate place names. More a linguistic than a literary category, names of cities, countries, rivers are a good example of a concrete research aspect of literary texts that is actually part of current research, such as in literary geography (Piatti, 2008). By the way, I have marked a total of 91 place names, which are to be entered here as 100%.

Quick Reading
Besides Close and Distant Reading there is of course a third variant (and there might be more). A skimmed, inaccurate or fast reading, in which the text is captured in its entirety, but not in all details. I call it “Quick Reading”, to be able to grasp it here somehow conceptually. In part, however, this fast, skimming reading outside of the Digital Humanities is also called Distant Reading.
The Quick Reading Self-Test
The step listed here as second was actually my first. In a spontaneous idea, I started to read and write down all place names in a table. This was done on the sofa, in the subway or wherever I just found a few minutes of time. The result was that of the 91 place-names found later during the Close Reading, I had annotated 67, about 74%. This admittedly not very good rate can of course be explained by a lack of care. But this alone does not explain it.
A question of interpretation
A second problem with what I call “Quick Reading” is that when you read and annotate categories spontaneously, they are often not precise enough. Even with a seemingly precise category such as location, there are always blurs. An example such as “he lives at Rothenbaumchaussee 71 near Hamburg University” can be considered as one place name or two. If, for example, you are interested in linking places with cultural significance, you might make two notes here: “lives “+ “Rothenbaumchaussee” and “Hamburg” + “University”. However, an interpretation as a reference to a place in which wohnt/Rothenbaumchausse/Hamburg/University is linked as a whole is also plausible. In the end, it definitely is a matter of perspective.
Advantages of Quick Reading
A major advantage of “Quick Reading” over Distant Reading is that, most of the times, nothing is wrongly marked (in the digital humanities, this would be called annotating false positives), but only occurrences of a category are overlooked (false negatives). Thus, not all mentions are recorded, but at least nothing is marked that is not really a place name. Also, this technique is quite fast and can handle medium-sized corpora instead of small amounts of text.
A disadvantage of Quick Reading
Besides the passages that actually fall into your analysis category, but which “slip through” to you, there is another disadvantage of the Quick Reading. If you edit an entire corpus in this way, it can be assumed that a learning effect will occur in the course of reading. In the end, this will result in the first data sets being of lower quality than the last ones. Even if one does not make the positive assumption that the learning curve will continue to rise, one can still expect that other characteristics will always slip into the focus of attention and thus one can at least speak of a certain heterogeneity of the data sets.
Distant Reading
Distant Reading is a term coined by Franco Moretti, who states in his book of the same name that literary scholars have probably not yet done research on more than 1% of all literary texts (Moretti, 2013). To be able to look at the rest, the 99%, what Moretti calls “the great unread”, we would have to learn not to read (Moretti, 2013). Because large amounts of text can only be viewed if we build up a not inconsiderable distance to the texts.
Here I will test only one of many distance reading methods, namely Named Entity Recognition. In my last article I already briefly introduced the Stanford Named Entity Recognizer (Finkel, Genager and Manning, 2005). StanfordNER recognizes with an accuracy of about 60-70% entities in texts, i.e. clearly identifiable entities such as places, organizations or persons. According to my understanding of place names as occurrences of concrete place names, the tool correctly recognized 53 entities, about 58%. This is slightly less than the 60-70% recognition accuracy that the tool achieves on average.
A possible explanation is the relatively narrow interpretation of the location category. For example, the phrase “in the gutter”, which is recognized by the tool, is not a place name, so it does not fall into my category. But it is indeed also plausible to interpret it as a place, because something can be located there. Another possible explanation is the domain for which the tool was optimized. StanfordNER was developed for factual texts and therefore achieves comparatively poor results as an “out of the box” solution for literature analysis (Jannidis et al., 2015). In any case, the Quick Reading brings me altogether to far more correctly marked places.
The great advantage of Distant Reading – the time saving
Of course, one has to praise and marvel that StanfordNER needed about 4 minutes to read the whole text (about 700 pages), while I spent about two weeks reading it and did not even annotate 1/7 of the text. I read about 15 minutes every day and if I calculate my whole reading time, I must confess I needed about 52 times longer than the software. So, with large corpora, the time factor should definitely be taken into account when deciding on a methodology.
The big disadvantage of Distant Reading – the incorrect recognition
In my sample, the StanfordNER incorrectly marked entities as places 33 times (false positives), which corresponds to 34% of all passages annotated as places. This error rate is quite high in my eyes. However, it is relatively easy to correct by simply editing the list of found entities. This way you will use up some of the time the tool has given you. Depending on the size of the corpus, this reworking of the database may take a reasonable amount of time. A second possibility offered by tools like StanfordNER is to work on the optimization of the software for your own domain. With a machine learning tool such as StanfordNER, this can be done through a separate training process.
Scalable Reading – the solution?
Besides Close and Distant Reading there is another method, Scalable Reading (Mueller, 2013). Scalable Reading is a fusion of Close and Distant Reading, the term inspired by Google earth’s possibilities of zooming in and out and the associated change of perspective. Müller, who coined the term, describes how zooming out from close to distant or non-reading makes more and more context visible that is included in analyses (Mueller, 2020). Especially zooming within an analysis project can create a lot of dynamics, the frequent changes of perspective can bring you very close to a corpus in the end.
If the idea of the scalar is combined with the idea of a combination of methods (in the sense of a mixed methods approach), a further project setting becomes conceivable. In order to be able to handle a medium to large corpus and still go very deep into the topic, partial corpora can be formed. A small core corpus can be handled with Close Reading. A medium-sized expansion corpus can be handled with Quick Reading to give an indication whether the phenomenon under consideration also applies to other texts.
The data created in this way can possibly even be used to train the Distant Reading Tool, which can then be applied to a frame corpus that is rather peripheral to the project. In this way, you not only set a clear focus for your project, which you look at very closely, but also get a feeling for whether your analysis is transferable.
How much distance to the text can a literary analysis cope with?
So you can see that the question of the distance you should or can reasonably maintain when analysing your texts is ultimately a question of weighing up. Up to 10 texts can be dealt with in a larger project such as a dissertation by close reading. 10 – 50 or, if you read very fast, maybe even up to 100 texts can be managed in a project period of about three years with Quick Reading. Distant Reading methods are required for a corpus of 100 texts at the latest. Maybe Scalable Reading would also be a great way for you to get in and out of your texts and thus combine text and context knowledge.
Translated with www.DeepL.com/Translator (free version)
[cite]
Bibliografie
Finkel, J. R., Genager, T. and Manning, C. (2005) ‘Incorporating Non-local Information into Information Extraction Systems by Gibbs Sampling. ’, in. 43nd Annual Meeting of the Association for Computational Linguistics (ACL 2005), Michigan.
Jacke, J. (2018) Manuelle Annotation, forTEXT. Available at: https://fortext.net/routinen/methoden/manuelle-annotation (Accessed: 22 June 2020).
Jannidis, F. et al. (2015) ‘Automatische Erkennung von Figuren in deutschsprachigen Romanen ’, in. DHD 2015, Graz.
Moretti, F. (2013) Distant Reading. London: Verso.
Mueller, M. (2013) Morgenstern’s Spectacles or the Importance of Not-Reading., Scalable Reading. Available at: https://scalablereading.northwestern.edu/2013/01/21/morgensternsspectacles-or-the-importance-of-not-reading/. (Accessed: 23 June 2020).
Mueller, M. (2020) Scalable Reading, Scalable Reading. Available at: https://sites.northwestern.edu/scalablereading/2020/04/26/scalable-reading/.
Piatti, B. (2008) Die Geographie der Literatur. Göttingen: Wallstein.
Schumacher, M. (2018) WebAnno, forTEXT. Available at: https://fortext.net/tools/tools/webanno (Accessed: 22 June 2020).
Schumacher, M. (2019) CATMA, forTEXT. Available at: https://fortext.net/tools/tools/catma (Accessed: 22 June 2020).
You May Also Like
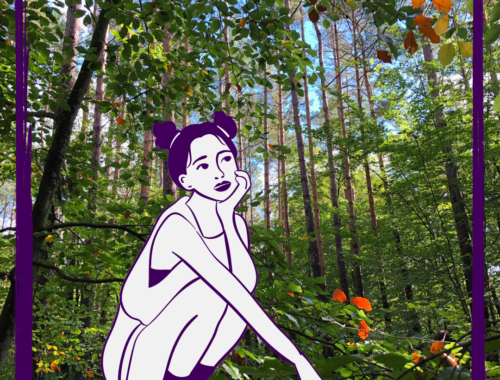
“Critical Digital Humanities”, A commentary
October 22, 2020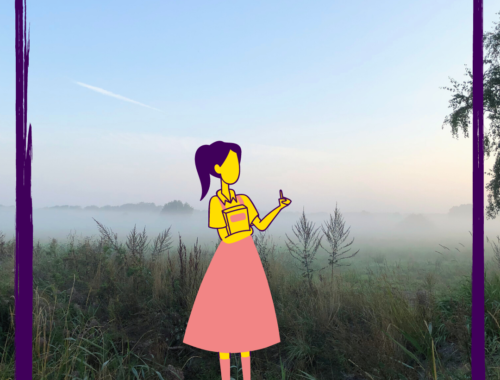
THE good book – what could that possibly be?
November 5, 2020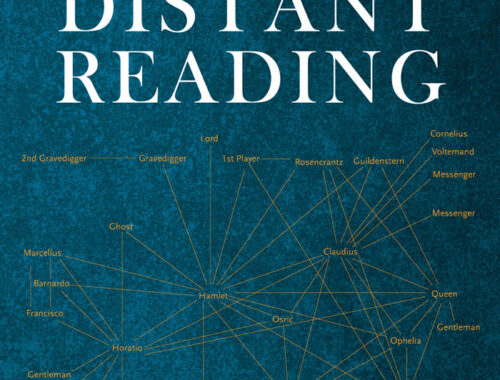